Functional traits are characteristics of organisms that are strongly connected to their fitness, or ability to reproduce and pass on their genes. In phytoplankton, cell size is one example, because it influences how quickly a cell can take up nutrients from its surroundings. By examining where and when cells of different sizes are found, we can better understand how the environment shapes the best size 'strategies'. Faster nutrient uptake rates may be most important in environments that experience occasional pulses of nutrients. This simple picture is complicated by trade-offs between traits, evolutionary constraints that prevent species from reaching the ideal trait value, and environments that are complex i.e. they have multiple dimensions to account for. Understanding these aspects of a community allows us to mathematically model how they will change when the environment changes, which improves our ability to forecast ecological and evolutionary dynamics.
My work focuses on a) identifying how changes in the environment across time and space drives changes in functional traits, b) characterizing the complex, multi-dimensional nature of fitness across important environmental gradients, and c) understanding how predictable phytoplankton communities are. To this end, I have worked on a number of projects relating to phytoplankton ecology, which I briefly describe below.
My work focuses on a) identifying how changes in the environment across time and space drives changes in functional traits, b) characterizing the complex, multi-dimensional nature of fitness across important environmental gradients, and c) understanding how predictable phytoplankton communities are. To this end, I have worked on a number of projects relating to phytoplankton ecology, which I briefly describe below.
1) The predictability of natural phytoplankton communities
How predictable are phytoplankton? Somewhat embarrassingly, we don't really know. Phytoplankton species may experience a new generation every day, under the right conditions. But when we study communities in natural systems, we miss a great deal of the picture because we measure them once every 2-4 weeks, roughly comparable to measuring forests every 200-500 years. If we are to improve earth systems models (that help forecast climate change) by including more realistic biology, we need to make reasonable forecasts on the range of decades to centuries.
I worked with Francesco Pomati at Eawag in Switzerland as a postdoc to address this. We used high-frequency scanning flow cytometry data (generated by the Cytobuoy) and long-term datasets to quantify predictability over timescales of hours to a decade. I found that basic machine learning techniques are an excellent fit for the multidimensional complexity of field datasets. Excitingly, we found that (i) phytoplankton communities are highly predictable, (ii) despite simultaneous changes in several environmental dimensions, we are able to disentangle how each is independently (to use the term loosely) affecting the phytoplankton, and (iii) these environmental dependencies point towards clear trade-offs that will help us develop better mechanistic models to forecast the future.
As part of this work, I developed semi-automated machine learning approaches to clean and analyse flow cytometry datasets. We have made resources for these methods are publicly available on Github and Zenodo (Example dataset, R code). In the future, we hope to apply these methods in real time to monitor natural communities in the field.
Collaborators: Francesco Pomati, Simone Fontana, Marta Reyes, Michael Kehoe
Papers:
1. The predictability of a lake phytoplankton community, over time-scales of hours to years [pdf]
2. Quantifying cell densities and biovolumes of phytoplankton communities and functional groups using scanning flow cytometry, machine learning and unsupervised clustering. [pdf]
How predictable are phytoplankton? Somewhat embarrassingly, we don't really know. Phytoplankton species may experience a new generation every day, under the right conditions. But when we study communities in natural systems, we miss a great deal of the picture because we measure them once every 2-4 weeks, roughly comparable to measuring forests every 200-500 years. If we are to improve earth systems models (that help forecast climate change) by including more realistic biology, we need to make reasonable forecasts on the range of decades to centuries.
I worked with Francesco Pomati at Eawag in Switzerland as a postdoc to address this. We used high-frequency scanning flow cytometry data (generated by the Cytobuoy) and long-term datasets to quantify predictability over timescales of hours to a decade. I found that basic machine learning techniques are an excellent fit for the multidimensional complexity of field datasets. Excitingly, we found that (i) phytoplankton communities are highly predictable, (ii) despite simultaneous changes in several environmental dimensions, we are able to disentangle how each is independently (to use the term loosely) affecting the phytoplankton, and (iii) these environmental dependencies point towards clear trade-offs that will help us develop better mechanistic models to forecast the future.
As part of this work, I developed semi-automated machine learning approaches to clean and analyse flow cytometry datasets. We have made resources for these methods are publicly available on Github and Zenodo (Example dataset, R code). In the future, we hope to apply these methods in real time to monitor natural communities in the field.
Collaborators: Francesco Pomati, Simone Fontana, Marta Reyes, Michael Kehoe
Papers:
1. The predictability of a lake phytoplankton community, over time-scales of hours to years [pdf]
2. Quantifying cell densities and biovolumes of phytoplankton communities and functional groups using scanning flow cytometry, machine learning and unsupervised clustering. [pdf]

2) Adaptation to temperature across the world's lakes and oceans: present patterns and future consequences
Rising temperatures are going to alter marine ecosystems and food webs, but how temperature influences phytoplankton today is poorly understood. To address this, I've collaborated with Colin Kremer to show that phytoplankton from pole to pole, in both lakes and oceans, are adapted to their local temperature conditions. Small amounts of warming in the tropics will make it uninhabitable for many present species, unless they adapt (in the evolutionary sense). Our work suggests that even now, temperatures in the tropics have risen sufficiently that we should be seeing either evolutionary adaptation or local extinctions. This parallels findings in terrestrial insects and lizards, which all point towards tropical environments as being particularly sensitive to environmental change.
Collaborators: Colin Kremer, Elena Litchman, Chris Klausmeier
Papers:
1. A global pattern of thermal adaptation in marine phytoplankton [pdf]
2. Environment and evolutionary history determine the global biogeography of phytoplankton temperature traits [pdf]
3. Marine phytoplankton temperature versus growth responses from polar to tropical waters – outcome of a scientific community-wide study [pdf]
4. Swift thermal reaction norm evolution in a key coccolithophore species [pdf]
Rising temperatures are going to alter marine ecosystems and food webs, but how temperature influences phytoplankton today is poorly understood. To address this, I've collaborated with Colin Kremer to show that phytoplankton from pole to pole, in both lakes and oceans, are adapted to their local temperature conditions. Small amounts of warming in the tropics will make it uninhabitable for many present species, unless they adapt (in the evolutionary sense). Our work suggests that even now, temperatures in the tropics have risen sufficiently that we should be seeing either evolutionary adaptation or local extinctions. This parallels findings in terrestrial insects and lizards, which all point towards tropical environments as being particularly sensitive to environmental change.
Collaborators: Colin Kremer, Elena Litchman, Chris Klausmeier
Papers:
1. A global pattern of thermal adaptation in marine phytoplankton [pdf]
2. Environment and evolutionary history determine the global biogeography of phytoplankton temperature traits [pdf]
3. Marine phytoplankton temperature versus growth responses from polar to tropical waters – outcome of a scientific community-wide study [pdf]
4. Swift thermal reaction norm evolution in a key coccolithophore species [pdf]
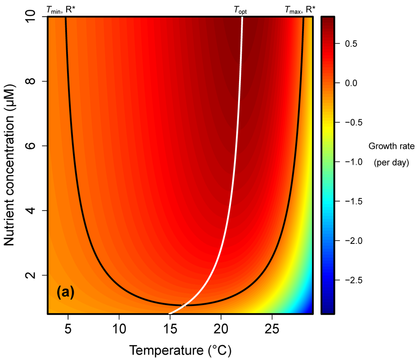
3) Interactive effects of multiple environmental factors on phytoplankton fitness
Equations that describe how fitness (or population growth rate) changes with some environmental variable are at the heart of models of population dynamics. Ecologists have successfully developed and tested these for individual environmental variables. But natural environments vary simultaneously in several dimensions, many of which are also changing because of human activity - temperature, pH, rainfall, nutrients, etc. Understanding how multiple factors interact to determine growth rate is therefore key to predicting population dynamics in natural environments and also a major challenge. In the phytoplankton, we know how growth rate changes across individual gradients in temperature, light, and nutrients, but have little idea of how simultaneous changes in even two of these influences it. My collaborators and I have worked to develop equations that describe the shape of this multidimensional fitness function, and then test these using experimental and field data. We published a model that makes novel predictions of how temperature and nutrients interact to influence growth (figure on right) and explored the consequences of this interaction.
Collaborators: Kyle Edwards, Elena Litchman, Chris Klausmeier, María Aranguren-Gassis, Colin Kremer
Papers:
1. Phytoplankton growth and the interaction of light and temperature: A synthesis at the species and community level. [pdf]
2. Temperature-nutrient interactions exacerbate sensitivity to warming in phytoplankton [pdf]
Equations that describe how fitness (or population growth rate) changes with some environmental variable are at the heart of models of population dynamics. Ecologists have successfully developed and tested these for individual environmental variables. But natural environments vary simultaneously in several dimensions, many of which are also changing because of human activity - temperature, pH, rainfall, nutrients, etc. Understanding how multiple factors interact to determine growth rate is therefore key to predicting population dynamics in natural environments and also a major challenge. In the phytoplankton, we know how growth rate changes across individual gradients in temperature, light, and nutrients, but have little idea of how simultaneous changes in even two of these influences it. My collaborators and I have worked to develop equations that describe the shape of this multidimensional fitness function, and then test these using experimental and field data. We published a model that makes novel predictions of how temperature and nutrients interact to influence growth (figure on right) and explored the consequences of this interaction.
Collaborators: Kyle Edwards, Elena Litchman, Chris Klausmeier, María Aranguren-Gassis, Colin Kremer
Papers:
1. Phytoplankton growth and the interaction of light and temperature: A synthesis at the species and community level. [pdf]
2. Temperature-nutrient interactions exacerbate sensitivity to warming in phytoplankton [pdf]